Introduction
LitGENE is an interpretable transformer-based model that integrates textual information through contrastive learning to refine gene representations. This approach leverages over a century of genetic research, employing summary data to predict the functions of genes.
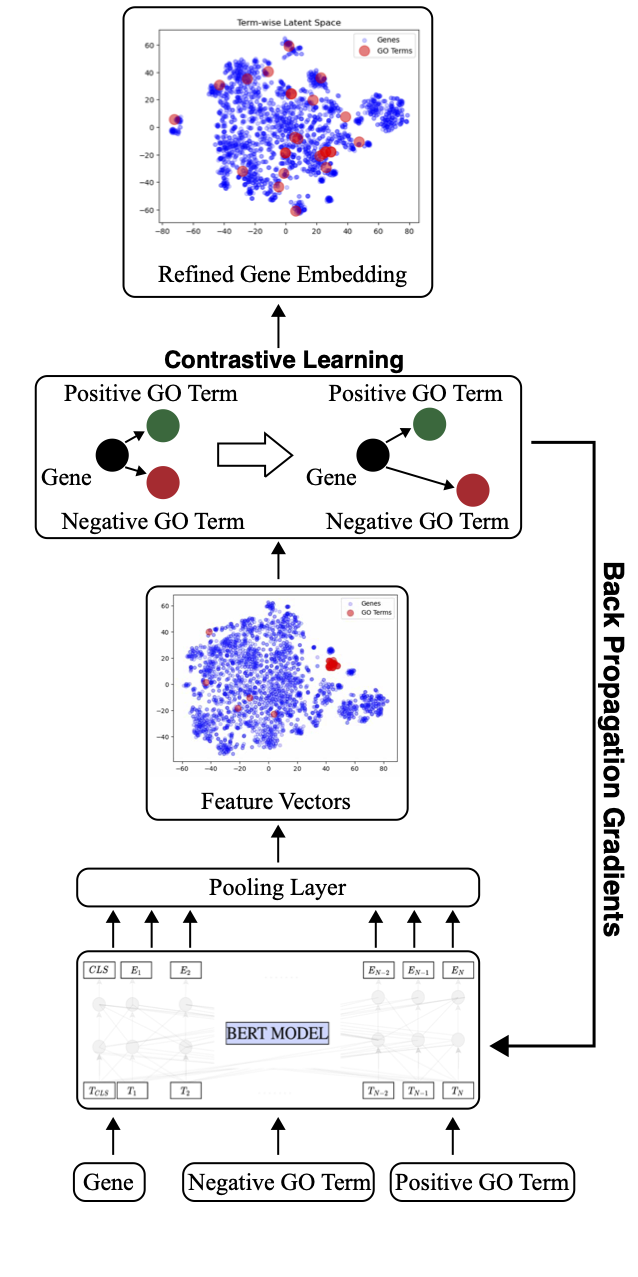
Figure 1: Overview of LitGENE Model
Technical Details
LitGENE incorporates a pre-trained language model augmented with Gene Ontology (GO) knowledge through contrastive learning. This infusion of information enriches gene representations, helping the model achieve superior performance in gene-related tasks.
The model uses BiomedBERT, a BERT-based text encoder specifically trained on biomedical text corpora. Contrastive learning draws the representations of semantically similar genes closer together in the embedding space while pushing apart those of dissimilar genes.
Key Features
- High Accuracy: LitGENE shows high accuracy across eight gene-related benchmarks, often outperforming task-specific models.
- Zero-shot Learning: Demonstrates robust zero-shot learning capabilities for unseen gene annotations, making it versatile for a wide range of predictive tasks.
- Interpretability: The model's interpretability and multimodal strategic approach to mitigating inherent data biases bolster its utility and reliability in biomedical applications where interpretability is paramount.
Webserver Functionality
The LitGENE webserver provides an interactive platform for users to explore gene-disease-drug associations. The webserver is built using Flask, a lightweight WSGI web application framework in Python, which makes it easy to scale and extend.
Users can input a gene, disease, or drug of interest and receive a summary based on extensive biomedical literature. The webserver also features advanced functionalities such as:
- Predictive Analytics: Using the integrated LitGENE model, the webserver predicts top associated genes, diseases, and drugs, providing similarity scores and links to external resources.
- Interactive Analysis: Users can analyze the importance of specific words in the input summary and find relevant citations from the literature, with results displayed dynamically.
- Visualization: The platform offers visualization tools to highlight the significance of words within the context of the input summary, aiding in better interpretability of results.
Applications
LitGENE has been evaluated on various gene-related tasks, such as solubility prediction, chromatin state prediction, dosage sensitivity, subcellular localization, and transcription factor target identification. It has shown superior performance, demonstrating its broad applicability in genomics and biomedical research. We recommend using LitGENE software (link) for these predictions. Currently, the webserver only supports predictions of related biological entities from input prompts.
Research and Development
LitGENE was developed by a team of researchers from the Department of Computer Science and the Comprehensive Cancer Center at The University of New Mexico. The team includes Ala Jararweh, Oladimeji Macaulay, David Arredondo, Olufunmilola M Oyebamiji, Luis Tafoya, Kushal Virupakshappa, and Avinash Sahu (Principal Investigator).
The findings affirm the complementary nature of unstructured text to structured databases in enhancing biomedical predictions while conscientiously addressing interpretability and bias for AI deployment in healthcare.
References
- Bruce Alberts. Molecular biology of the cell. Garland science, 2017.
- Haotian Cui, Chloe Wang, Hassaan Maan, Kuan Pang, Fengning Luo, and Bo Wang. scgpt: Towards building a foundation model for single-cell multi-omics using generative ai. bioRxiv, 2023.
- Guilliermo Owen. Values of games with a priori unions. In Rudolf Henn and Otto Moeschlin (eds.), Mathematical Economics and Game Theory, 1977.
- Thomas Stoeger, Martin Gerlach, Richard I. Morimoto, and Luı́s A. Nunes Amaral. Large-scale investigation of the reasons why potentially important genes are ignored. PLOS Biology, 16(9), 09 2018.
- Jingcheng Du, Peilin Jia, YuLin Dai, Cui Tao, Zhongming Zhao, and Degui Zhi. Gene2vec: distributed representation of genes based on co-expression. BMC Genomics, 20(1), 2019.
Contact
For more information, visit our website or contact us at info@avisahuai.com.